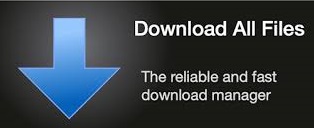
used the EEG to track ERPs associated with reward processing. The EEG data analysis of reward processing often relies on event-related potentials (ERPs), time-frequency analysis, and blind source separation (BSS). The gambling task is a well-established classic paradigm suitable for studying the cognitive processes of reward processing, which are usually investigated by EEG and fMRI. On the other hand, reward outcome corresponds to those operations triggered by the actual delivery of a reward, such as hedonic feelings, reward value updating, and behavioral reinforcement. Reward anticipation usually involves the cognitive operations that precede the arrival of a reward, including incentive evaluation, probability estimation, and motor preparation. A typical reward-processing progress is made up of two parts: the anticipation and the outcome phase, each of which involves several subprocesses that co-occur on different time scales. Yet, comprehending how the brain processes rewards still remains a non-trivial outstanding question. It is often used in mental health diagnoses as many psychiatric disorders are accompanied by certain deficiencies while processing rewards. Reward processing is central to emotional decision-making and risk-taking. Conclusion: This work sheds light on the reward-processing progress, provides a deeper understanding of brain function, and opens a new avenue in the investigation of neurovascular coupling via CMTF. Meanwhile, regions such as the superior orbital frontal gyrus and anterior cingulate cortex are activated upon winning stimuli, whereas the inferior frontal gyrus, cingulate cortex, and medial superior frontal gyrus are activated upon losing stimuli. It is found that regions including the orbitofrontal cortex and insula are activated for both winning and losing stimuli.
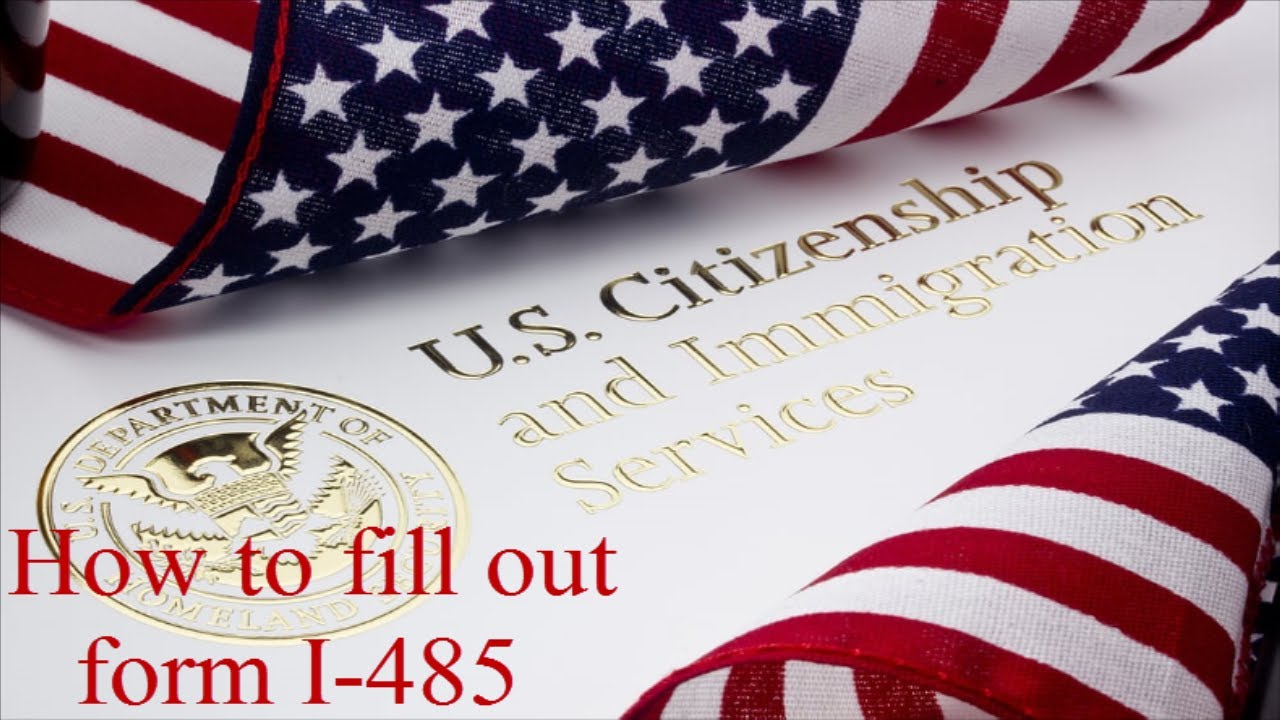
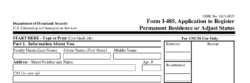
Results: The results clearly show that not only are the regions of interest (ROIs) found in other literature activated, but also the olfactory cortex and fusiform gyrus which are usually ignored. Finally, graph-structured clustering is used to select the most appropriate model according to four quantitative indices. Next, the EEG and fMRI data are jointly decomposed into a superposition of multiple sources characterized by space-time-frequency profiles using coupled matrix tensor factorization (CMTF). Methods: First, the single-subject EEG data are represented as a third-order spectrogram tensor to extract frequency features. Here, we report the first attempt for a simultaneous electroencephalography (EEG)–functional magnetic resonance imaging (fMRI) study in a gambling task by utilizing tensor decomposition. Background: It is crucial to understand the neural feedback mechanisms and the cognitive decision-making of the brain during the processing of rewards.
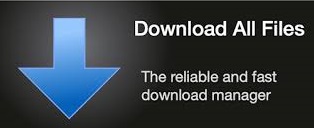